Dr. Martin Hofstetter is Head of E-Mobility and Alternative Powertrains at the Institute of Automotive Engineering (TU Graz). At Dritev, he talks about the agile development of electric powertrains using AI-based design optimization.
Making Product Development Agile
Artificial Intelligence as a Game Changer for E-drive Development
The demands on electric drivetrains are high: energy efficiency, space optimization, sustainability, performance, costs, service life and much more – and all this under high time pressure during development. But how can this multitude of factors be combined in a balanced technical design, while at the same time meeting ambitious schedules? Dr. Martin Hofstetter, Head of E-mobility and Alternative Powertrains at the Institute of Automotive Engineering, Graz University of Technology, will be presenting a solution at the VDI Congress DRITEV 2025 on 9 and 10 July 2025 in Baden-Baden: an AI-based method for the agile design optimization of electric powertrains. In our interview, he describes the special features and advantages of the technology.
Dr. Hofstetter, what impetus for agile, AI-based product development can Dritev 2025 participants expect from you?
Dr. Martin Hofstetter: Today, we are dealing with product development that is hard to beat in terms of complexity. Electric drivetrains have to be energy-efficient, powerful, cost-effective, sustainable and modular at the same time – and still fit into a strictly defined installation space. This multitude of requirements leads to conflicting objectives that can no longer be met within tight time frames using conventional approaches.
Our approach at TU Graz is based on an AI-supported method that makes it possible to systematically penetrate this complexity and make optimized design decisions in a significantly shorter time. The key message here is that we can use AI-based development tools to make complexity manageable and achieve a new level of agility in drive development.
What can your method do that traditional development processes cannot?
Dr. Martin Hofstetter: Conventional processes usually run sequentially, are iterative and time-consuming. If requirements or priorities change in the course of development – which happens regularly – the process has to start all over again. With our method, on the other hand, such changes can be taken into account in real time. To do this, we use evolutionary optimization algorithms combined with self-learning AI systems. In this way, thousands and thousands of variants of a drivetrain can be run through digitally and analyzed with regard to target variables such as costs, efficiency or sustainability – all within 24 hours. The result is a flexible, data-based decision-making system that opens up new technical solutions at the touch of a button. This also makes it possible to consider factors influencing development that go far beyond purely technical aspects. For example, objectives from engineering, costing and sustainability are weighed up together on a “digital table” instead of being evaluated sequentially and iteratively across several specialist departments in the traditional development process.
How exactly does this “evolutionary” process work?
Dr. Martin Hofstetter: The idea is based on natural selection: good solutions are retained, bad ones are discarded. In the digital world, this works in such a way that the algorithm starts with a large number of possible design variants. These “evolve” over many virtual generations – always in line with the target values specified by the user. This results in design proposals that are optimally tailored to the respective requirement profile. This process is highly efficient, robust and comprehensible – and in combination with physically based models, it is also realistic and verifiable. We have further developed these evolutionary algorithms for our requirements and combined them with self-learning AI systems – this reduces the calculation time enormously and allows us to solve highly complex problems that would otherwise not be solvable in a practical time.
What role do these physical models play?
Dr. Martin Hofstetter: A central one. This is because we deliberately do not work with simplified mathematical system models, but with digital representations of real component geometries. This means that every result that our system provides is not only theoretically optimal, but also corresponds to a concrete, physically feasible product solution – including gear geometry, bearing selection, inverter arrangement and so on. This makes validation much easier. Our results can be integrated directly into existing CAD and simulation processes – for detailed strength testing or detailed cost calculations, for example. This means that what results from agile development is much more than just an idea, but a technical design that can be implemented and verified.
Does this also mean that your method is not just theory, but is already being put into practice?
Dr. Martin Hofstetter: Exactly. The method was originally developed as part of an industrial project together with a Tier 1 supplier – incidentally, this was also the subject of my dissertation. The technology has been established there in the real development process for several years and has proven itself many times over. And that is important to us: we do not conduct basic research in an ivory tower but develop tools that work in industrial practice.
What exactly does the system deliver in the end – and how does this help with decisions?
Dr. Martin Hofstetter: The central result is a so-called Pareto front. It shows all technically feasible trade-offs between competing objectives – for example between costs and energy efficiency or CO2 balance and installation space. The decision-makers in the development team can then select a solution that meets their priorities, even with the direct involvement of the customer. And with a high level of transparency and traceability. For each point on the Pareto front, there is a complete design with detailed technical evaluation that is suitable for the installation space. This means that the decision is made on the basis of the overall product performance instead of having to deal with detailed decisions yourself. In addition, the structured approach and comparison with alternative solutions means that it can be confidently demonstrated that the best solution has actually been selected in the huge solution space.
How do engineers react to this new form of support?
Dr. Martin Hofstetter: Initially with curiosity – and admittedly sometimes with a little skepticism. But we see that this skepticism quickly turns into enthusiasm when the first results are available. Because one thing is clear: the method does not replace engineers – it relieves them. Especially in the early phases, for example in the case of a development request with tight deadlines. There are often only a few weeks to deliver a concept. Our method creates a reliable basis for decision-making within a very short time. The engineers can then concentrate on what they do best: working out the details with all their experience. All in all, you simply achieve a sense of achievement more quickly.
How agile is the system when requirements change?
Dr. Martin Hofstetter: This agility is a decisive advantage. Requirements change frequently – be it due to new legal requirements, market changes or internal decisions. The method allows such changes to be incorporated and calculated immediately. The AI generates new solution proposals based on the new framework conditions within a day. This is not only faster, but also significantly more efficient than conventional product adaptations. As a result, this means a real competitive advantage for companies that rely on this AI-based technology at an early stage. Ultimately, customers and partners also benefit from this agility, and I am convinced that the market will demand this level of agility in the future.
Are there limits to this approach? What is not yet possible – or where do you see challenges?
Dr. Martin Hofstetter: Of course there are limits. AI can only work with what we make available to it as the state of the art and as a database. This means that new technologies for electrical machines or semiconductors, for example, must first be mapped in the digital models. Another challenge is anchoring them in existing company processes – because innovative tools often come up against organizational inertia. Especially in difficult economic times, people tend to save on investments in new methods – but that would be a mistake in my view.
Why should we invest in AI-supported development now instead?
Dr. Martin Hofstetter: Because now is exactly the right time to position yourself technologically for the future. The market is highly dynamic and international competition – from China, for example – is becoming ever more intense. If you want to survive, you must develop faster, more flexibly and more efficiently. AI-supported methods offer precisely this opportunity: they help to make the right decisions faster, save development time, minimize risks – and ultimately reduce costs.
What's next for your method? Is it also available to other interested parties from the industry?
Dr. Martin Hofstetter: Absolutely! The technology is based at Graz University of Technology and we own the intellectual property. We currently have cooperation partners from industry – and we are open to further partnerships, including for other technical products and in other sectors. After all, the basic idea of quickly finding optimal solutions based on AI in a highly complex requirements space can also be easily transferred to other fields of application.
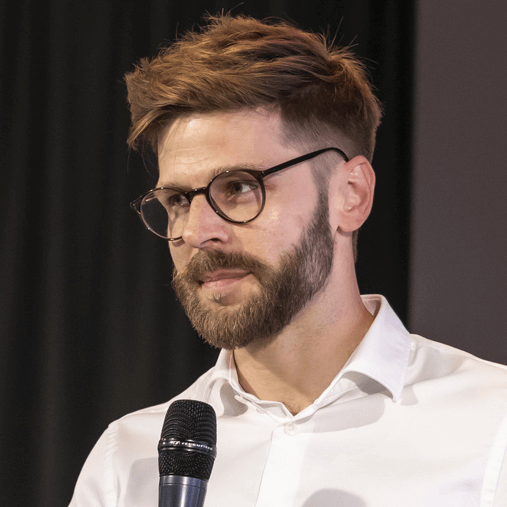
Image: private